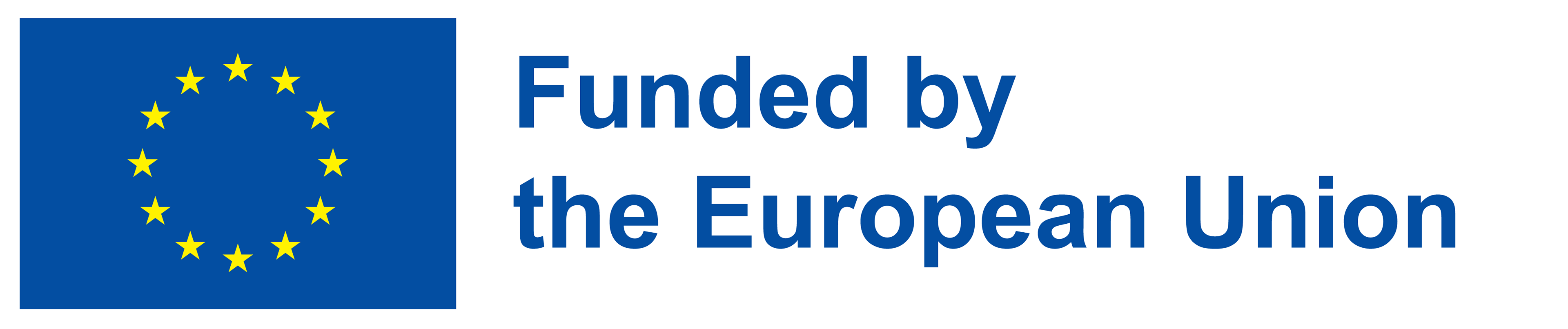
Ongoing
Collaborative Project with multiple EU partners, coordinated by Prof. Helen Gika and PD Dr. Michael Witting
Funded by: European Union - Project Number 101073062
Project Description
Metabolomics provides a real-time view of the metabolic state of the examined samples. The past decade the field showed strong growth, however limitations intrinsic to the field hinder further application in epidemiology level. Key obstacles include: variety of analyte molecular structures, slow marker identification, large differences in concentrations, poor validation, incomplete combination of data from different analyses and fragmentation of research. The consortium brings together scientists from different complementary disciplines and sectors to collaborate and set a research training network, combining infrastructure experience, knowledge and skills. The research scope is to identify the source of problems that hinder development, and recommend measures to overcome these. Training through
research will promote a new generation of omics researchers. Networking, joining forces via secondments will enhance research productivity transfer of knowledge. The project will train 10 ESRs in work-packages aiming toward improvement of design of experiment, harmonization of analytical methods, improved Data Mining and biochemical pathway analysis and translational research. Application will be in the study of blood metabolome of exhaustive physical exercise.
We aim to study sample stability & preparation (including blood and alternative forms such as dried blood spots), biomarker identification, exploitation of multiple datasets, promote standard procedures, develop robust pipelines, develop and implement machine searchable notations of metadata, central database for data storage, compare datasets, automate cross-laboratory data combination, develop novel algorithms for multidimensional data mining and reconstruct biochemical pathways. The overall goal is to train the ESRs in cutting edge metabolomics research and at the same time provide proof of concept of democratizing metabolomics by the use of patient centric sampling.
research will promote a new generation of omics researchers. Networking, joining forces via secondments will enhance research productivity transfer of knowledge. The project will train 10 ESRs in work-packages aiming toward improvement of design of experiment, harmonization of analytical methods, improved Data Mining and biochemical pathway analysis and translational research. Application will be in the study of blood metabolome of exhaustive physical exercise.
We aim to study sample stability & preparation (including blood and alternative forms such as dried blood spots), biomarker identification, exploitation of multiple datasets, promote standard procedures, develop robust pipelines, develop and implement machine searchable notations of metadata, central database for data storage, compare datasets, automate cross-laboratory data combination, develop novel algorithms for multidimensional data mining and reconstruct biochemical pathways. The overall goal is to train the ESRs in cutting edge metabolomics research and at the same time provide proof of concept of democratizing metabolomics by the use of patient centric sampling.
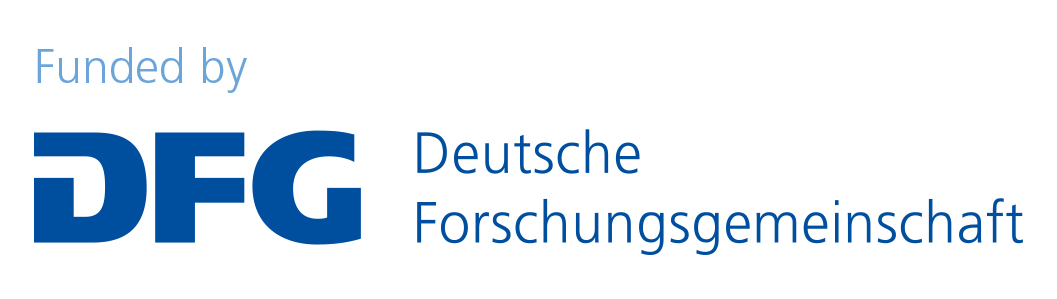
Ongoing
Transferable retention time prediction for Liquid Chromatography-Mass Spectrometry-based metabolomics
Collaborative Project with
- Prof. Dr. Sebastian Böcker, Friedrich-Schiller-Universität, Jena, Germany
Funded by: Deutsche Forschungsgemeinschaft (DFG) - Project Number 425789784
Project Description
Metabolite identification still represent the major bottleneck in metabolomics. Liquid Chromatography-Mass Spectrometry (LC-MS) is the currently most employed analytical technique in untargeted metabolomics. Currently, less than 10% of spectra in a typical untargeted experiment can be annotated. Therefore, there is a strong need for improved tools for metabolite identification. While mass alone cannot identify molecules, tandem MS yields fragmentation spectra which can be used for structural elucidation. Recently, in silico approaches have been developed and are increasingly used by the metabolomics community, that allow to search in molecular structure databases such as PubChem and ChemSpider. Such structure databases are many orders of magnitude larger than any spectral library and, hence, have a much wider coverage of molecular structures. But even identification by tandem MS will result in numerous spurious identifications. To improve identification quality, two independent parameters, e.g. mass and retention time of a chemical reference standard have to be reported. Today, retention time is mainly used at a later stage of the identification pipeline, and mainly based on comparison with chemical reference standards. However, it would clearly be beneficial if retention times were used at an early stage, in particular for in silico methods; here, we could filter candidates or, even better, modify the scores of candidates based on comparing predicted and observed retention times.This project aims to make better use of retention times for the identification of small biomolecules in LC-MS based untargeted metabolomics, using transferable retention time prediction. Prediction will be based on a two-step approach. First, Machine Learning will be used to predict retention order numbers for give molecular structures; training will be based on an extensively curated collection of retention time data from public available datasets, as well as systematic in-house measurements for reference metabolite standards. In contrast to its mass, retention time is not a feature of a metabolite, but of the combination of metabolite, stationary and mobile phase. Therefore, we will use properties of the employed chromatographic system in addition to molecular fingerprints of metabolites for machine learning. In the second step, retention order numbers will be mapped to retention times, using known and identified substances as anchors of the mapping. Retention order and retention time prediction will be used to filter false positive reaction pairs, and applied to an independent biological dataset from C. elegans secondary metabolism.All curated and acquired data, open-source software for prediction of retention order and retention times will be made freely available to the metabolomics community. Finally, retention time prediction will be integrated into the CSI:FingerID scoring in order to improve its metabolite identification rates.
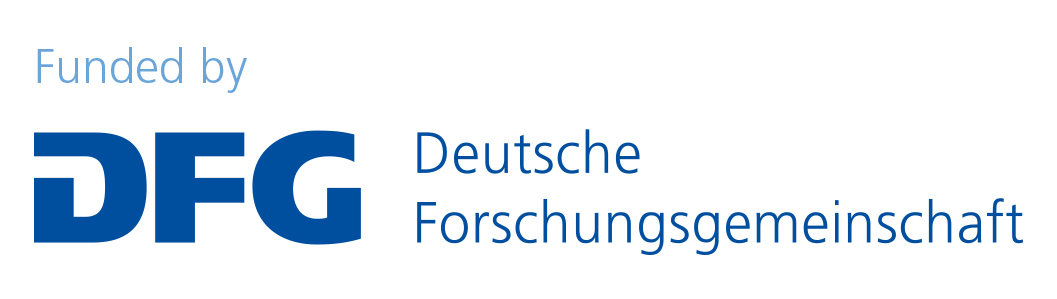
Finished
MetClassNet: new approaches to bridge the gap between genome-scale metabolic networks and untargeted metabolomics
Collaborative Project with
- Dr. Steffen Neumann, Leibniz-Institut für Pflanzenbiochemie, Halle, Germany
- Dr. Fabien Jourdan, INRA ToxAlim, Toulouse, France
- Dr. Reza Salek, International Agency for Research on Cancer, Lyon, France
Funded by: Deutsche Forschungsgemeinschaft (DFG) - Project Number 431572533
Project Description
Metabolism is a key biological process which is modulated in living organisms in response to environmental exposure, genetic variations and diet. Understanding metabolism is essential to improve plant performance, nutritional content, and to understand Human health and well-being. The metabolic response can be complex, involving hundreds to thousands of small molecules (metabolites) connected by thousands of biochemical reactions. Together, they constitute a dense network, in its entirety often called Genome Scale Metabolic Network (GSMN). Within this context, metabolomics is a cornerstone approach to experimentally observe changes in the metabolome (set of metabolites). One of the main analytical platforms to measure the metabolome is Mass Spectrometry (MS) which is often coupled to separation methods (e.g. Liquid Chromatography, LC-MS). Even though the technology is advancing rapidly, several challenges remain for widespread adoption of metabolomics. Metabolite identification remains one of these challenges. Nevertheless, experimentally obtained data and in silico generated GSMN overlap only partially and are generally not studied simultaneously. In MetClassNet, we hypothesis that these difficulties could be overcome by designing new data structures and algorithms which will exploit the connectivity (network) between molecules. This integrative approach will boost the power of data analysis by unifying GSMNs and networks obtained from experimental data. Hence, MetClassNet will propose a new computational framework and novel methods to help with tackling main metabolomics challenges in data analysis and data interpretation. This framework will integrate information from experimentally derived information and GSMNs by bridging them using direct mapping, ontologies and chemical class information.At the end of the project, MetClassNet will offer the community an innovative tool set where it will be possible to go beyond table based analysis of metabolomics data by integrating (and not just exporting) them into a network system. To this end, MetClassNet will create novel algorithms and tools to mine these networks allowing to increase our knowledge of the metabolome. The developed framework will also ease the connection between metabolomics and GSMNs, hence allowing to fill the gaps in current databases of metabolic networks. Within MetClassNet project, we will showcase the benefit of the computational framework to address the study of metabolic modulations related to ageing, toxicology, cancer and nutrition. Finally, MetClassNet consortium will put the necessity of opening data, protocols and software to the community high in its agenda.